Representing Aotearoa New Zealand at the 2023 Australasian Leadership Computing Symposium
Earlier this month, NeSI team members and a small cohort of researchers from Aotearoa New Zealand gathered with science communities at the forefront of High Performance Computing (HPC), Artificial Intelligence (AI) and Data Science research for the 2023 Australasian Leadership Computing Symposium (ALCS).
Co-hosted by NeSI, NCI Australia, and the Pawsey Supercomputing Research Centre, ALCS took place at the Shine Dome in Canberra from 14-16 June 2023.
NeSI was keen to support representation and participation from Aotearoa New Zealand research communities at ALCS2023, so sponsored three early career researchers to attend and present: Fei (Travis) Dai, Joseph Guhlin, and Hannah Kessenich.
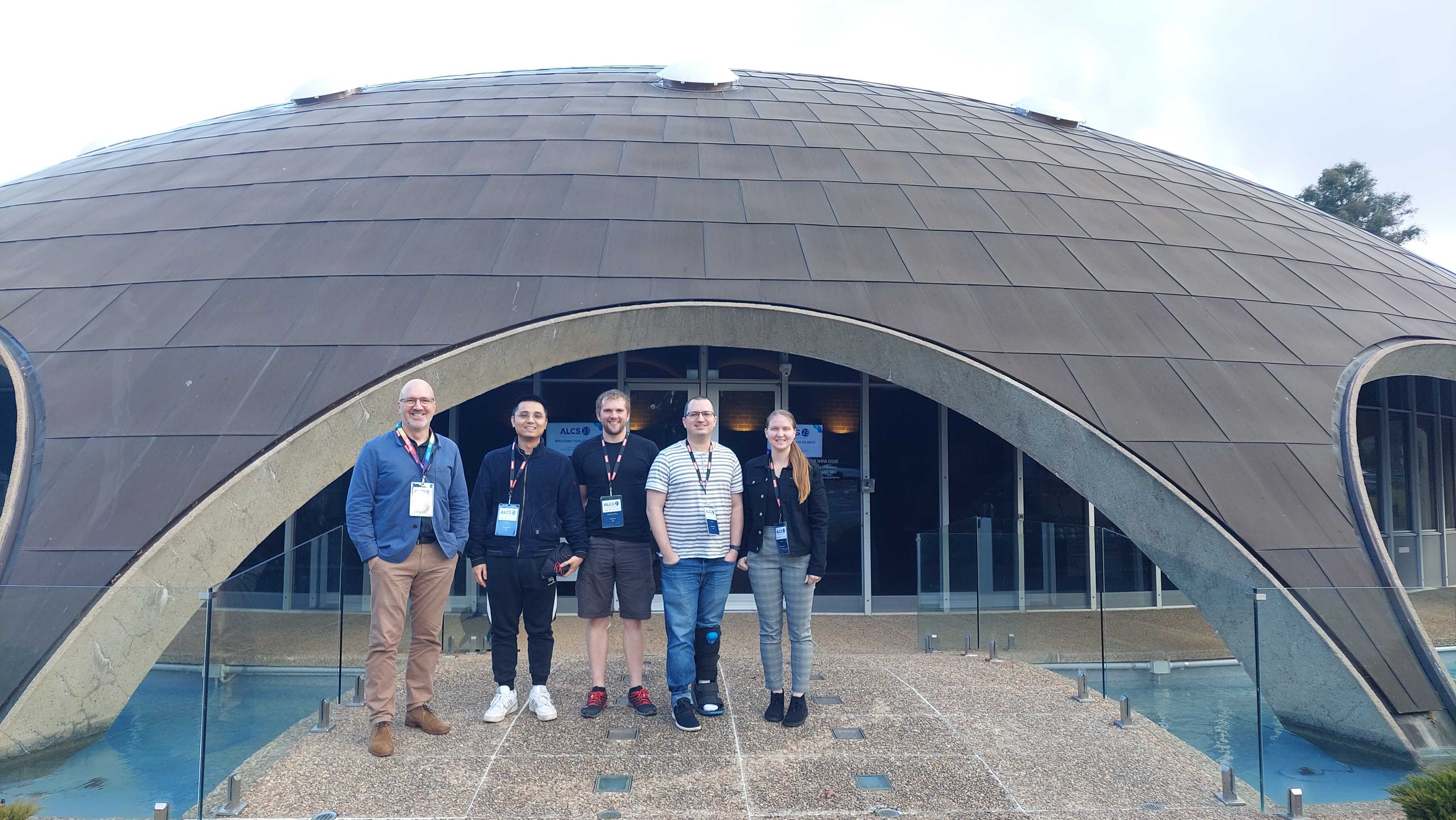
Read more about their ALCS talks below and stay tuned for a more in-depth recap of highlights from the event. We're aiming to sit down with Hannah, Fei, and Joseph in the coming weeks for a chat about their takeaways from ALCS 2023.
Hannah Kessenich
Talk title:
Improving Antarctic ozone prediction in a changing climate
Attribution:
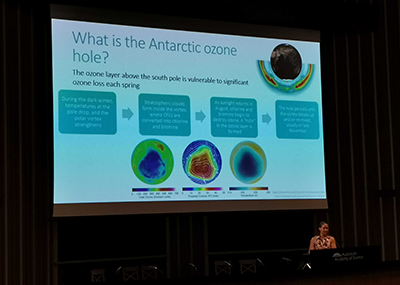
Objectives
The past three years (2020–2022) have witnessed record-large Antarctic ozone holes, despite expected ozone recovery since the Montreal Protocol. Our team has found evidence of a significant ozone decline (26%) since 2004 at the core of the Antarctic ozone hole during October, the springtime month when the hole reaches its maximum size. We have found evidence of possible new prediction mechanisms for Antarctic ozone which could improve ozone representation in climate simulations.
Methods
We apply regression-based methods on large satellite datasets encompassing Antarctic ozone behaviour over several decades. A range of analyses are performed to: 1) investigate regional trends in ozone levels and 2) explore potential new predictors for such trends.
Results
We have taken a detailed look at Antarctic springtime ozone as it evolves daily in altitude and latitude. Significant long-term variability, currently not well captured by model predictions, was found in polar ozone. Analysis of other atmospheric variables suggest this is driven by both chemical and dynamical drivers.
Conclusions
Antarctic ozone loss is known to influence surface temperatures, sea ice extent, and large climate patterns such as the driving force behind the winds that spread the recent Australian bushfires. Realistic representation of Antarctic ozone variability in climate simulations would allow for enhanced prediction of ozone recovery and its impacts on the climate system. To establish a new model representing Antarctic stratospheric ozone variability, we have identified new predictors to base the model on.
Joseph Guhlin
Talk Title:
The Hymenopteran Unified Gene Set: Reannotating 284 genomes
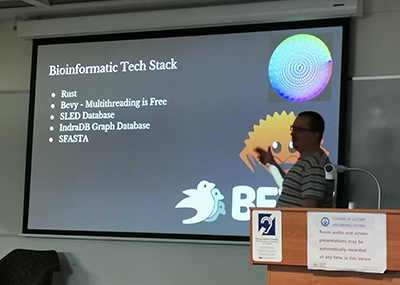
Genomics is now entering the era of ‘big data.’ Instead of a single species, there is a demand to compare across many species and sometimes even across populations found in different species. Traditional software development techniques for bioinformatics are rarely sufficient for handling large datasets. Genome annotation is necessary to identify the functional units of genomes; however, different methods and labs have different biases in the output. Genes are combinations and sets of open-reading-frames (ORFs) localized to genome regions.
As related species are derived from common ancestors, most of these genes have shared evolutionary histories. By improving genome annotation, we create higher-quality gene models, which can assist in conservation, plant and animal breeding and help answer scientific questions. I am taking ~284 genomes from Hymenoptera (ants, wasps, bees, and others) to reannotate. These high-quality, consistent gene models will help us identify eusociality’s evolutionary origins, improve common and uncommon venom genes, and help elucidate sensory genes, which can significantly impact agriculture (bees and pests).
To do this, I am finding similar ORFs and using graph algorithms to find directed cyclic graphs of ORFs between species, to stitch together the ORFs that have shared common ancestry, and use this to run gene prediction algorithms to annotate genomes of many hundreds, instead of individually rapidly. To do this, I use a game development engine’s Entity Component System (ECS) features, offering effortless multi-threading, high-speed, and memory management.
Fei (Travis) Dai
Talk Title:
WRHT: Efficient All-reduce for Distributed DNN Training in Optical Interconnect System
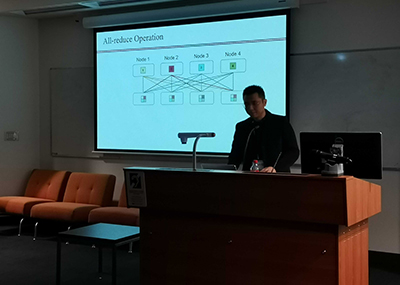
Communication efficiency plays an important role in accelerating the distributed training of Deep Neural Networks (DNN). All-reduce is the crucial communication primitive to reduce model parameters in distributed DNN training. Most existing all-reduce algorithms are designed for traditional electrical interconnect systems, which cannot meet the communication requirements for distributed training of large DNNs due to the low data bandwidth of the electrical interconnect systems.
One of the promising alternatives for electrical interconnect is optical interconnect, which can provide high bandwidth, low transmission delay, and low power cost. We propose an efficient scheme called WRHT (Wavelength Reused Hierarchical Tree) for implementing all-reduce operation in optical interconnect systems. WRHT can take advantage of WDM (Wavelength Division Multiplexing) to reduce the communication time of distributed data-parallel DNN training. We further derive the required number of wavelengths, the minimum number of communication steps, and the communication time for the all-reduce operation on optical interconnect. The constraint of insertion loss is also considered in our analysis.
Simulation results show that the communication time of all-reduce by WRHT is reduced by 80.81%, 64.36%, and 82.12%, respectively, compared with three traditional all-reduce algorithms according to our simulation results of an optical interconnect system. Our results also show that WRHT can reduce the communication time of all-reduce operation by 92.42% and 91.31% compared to two existing all-reduce algorithms running in the electrical interconnect system.