A new spatial model for estimating New Zealand soil carbon
Dr Stephen McNeill, Dr Allan Hewitt (Landcare Research), and Dr Andrew Manderson (AgResearch) lead a project that sought to build a model that estimates the total carbon content in the soil for every location over the landscape, as well as the uncertainty in the estimates.
This project is part of a larger programme of work funded by the New Zealand Agriculture and Greenhouse Research centre (NZAGRC) which aims to determine current quantities of soil carbon in New Zealand, how much additional carbon our soil is capable of storing, and methods for improved management of the soil resource. Carbon content is a major factor in the overall health of soil and we know that humans strongly influence the amount of carbon in the soil through losses induced, for example, by tillage and erosion, and gains sustained by re-vegetation and changes in land-use practise.
To build the model, the researchers assembled all the major soil carbon data sources from New Zealand, as well as a range of map layers thought to be associated with soil carbon. These layers describe climate and terrain, as well as soil and landscape. The aim was that the map layers could be used along with the soil carbon sample data to build a model that describes soil carbon in terms of the various map layers. It would then be possible to estimate soil carbon over the landscape using the collection of layers.
There are several problems with this conceptually simple approach. First, the large number of map layers available for use as predictors, as well as their possible interactions, makes the problem of finding the “best” selection of predictors very difficult. Second, available soil data are patchy; some areas are well covered by field measurements while other regions and soil types are relatively sparse in coverage. The disparity in sampling over the landscape causes problems in building a model for soil carbon, especially in respect of the uncertainty.
The researchers used a method from data mining (“boosting”) to find out the best set of map layers to predict soil carbon. Boosting is a method that can readily accept a large number of potential predictors of soil carbon, testing each predictor and their interaction in a systematic manner. Boosting has some disadvantages, however; the method can be quite slow and provides a model that is difficult to interpret. So the result from data mining was used as a starting point for a conventional statistical model-building exercise, but it was a crucial step that drastically reduced the time taken to develop the final model for soil carbon.
A critical step in the research was the development of a model for the uncertainty of the soil carbon estimates, which is the range of soil carbon values within which one might find the “true” value of soil carbon, given some set of climate, terrain, and other landscape conditions. Model uncertainty is essential if the uncertainty or confidence estimates are needed in downstream agricultural and climate change models that rely on these soil carbon estimates. This model is currently being used in a collaborative project with the University of Sydney to develop fine-spatial-scale models of soil carbon; this work is funded under the Global Research Alliance (GRA). The model has potential to be used by other GRA countries in future, particularly where soil carbon is required as an input to ecosystem models.
One key diagnostic step in the generation of the soil carbon stock model was to confirm the distribution of the model coefficients. One way that this can be done is to use a bootstrap analysis. Using this approach on a modern Windows machine this job was estimated to take about 21 days of computing time, which was not considered practical. Using the NeSI high performance computing platform called ‘Pan’ at the University of Auckland, we were able to substantially reduce this time by implementing the bootstrap analysis in parallel. We used R with the snow package to allocate the various bootstrap iterations to R tasks, assembling the data in the calling task on return. Using this approach, the job was carried out over less than a day of computing time, and the subsequent analysis of the results confirmed the appropriateness of the soil carbon model developed.
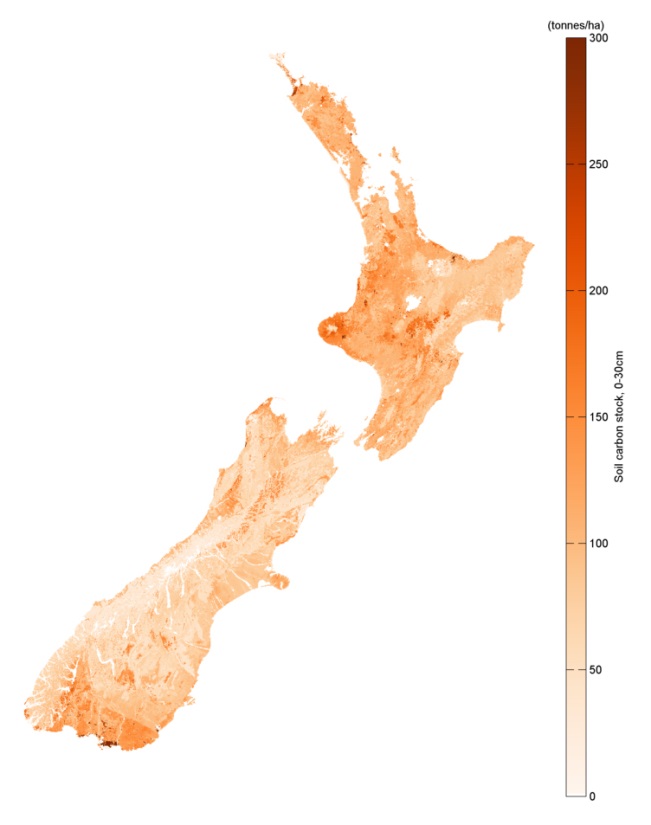
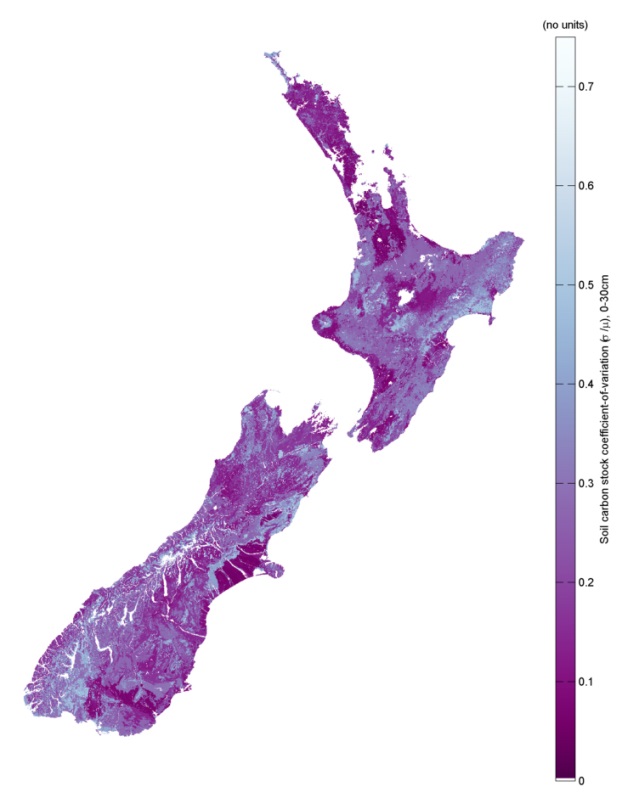