Modelling wind for Fiji’s green future
The Challenge:
Fiji targets to move away from fossil fuel reliance to 100 percent renewables for electricity generation by 2030 as committed by their Nationally Determined Contributions (NDCs) under the United Nations Framework Convention on Climate Change (UNFCCC). Wind farms could be an important step to achieving this goal. But, Fiji lacks quality long-term mesoscale wind-resource maps at applicable wind turbine hub-heights that can be used for the identification of potential wind farm sites and long-term time series wind resource data to model wind farms via a comprehensive wind-resource assessment.The Solution:
Use NeSI computing resources to power the state-of-the-art Weather Research and Forecasting (WRF) numerical weather predication model, using initial and boundary conditions from General Circulation Model analysis data via the dynamical downscaling methodology. These tools are helping researchers to map the recent decade wind resources at a high-resolution of 1 km × 1 km for the Fijian Islands.The Outcome:
Creation of a recent decade (2009 – 2018) high-resolution (1 km × 1 km) mesoscale wind resource map at 55 m elevation, and high-resolution hourly time series wind resource data at 1 km × 1 km resolution for decade-long for the whole of Fiji, even in locations where ground-based measurements are not available [1]. The modelling exercise revealed 40 high wind-resource areas, which were previously unknown with an estimated theoretical potential installed capacity of 1000 MW available for utility-scale wind power applications on Viti Levu and Vanua Levu [1]. This could attract government and industry investment.
Fiji’s 100 percent renewable energy target by 2030 requires a massive ramp up of renewable energies in the country. Because of its low cost and land usage, wind power will be a vital component for meeting this target.
In 2019, the world used 651 gigawatts of wind energy, making up roughly 5 percent of global electricity generation. At the moment wind energy makes up only 0.25 percent of Fiji’s energy production, with over half the country’s power running on hydropower. While hydropower is renewable, around 41% of the country still runs on petroleum. Hydropower alone can’t cut Fiji’s fossil fuels, so wind will be a vital future technology.
Kunal Dayal, a PhD student in Mechanical Engineering at the University of Auckland, is participating in a New Zealand Foreign Affairs and Trade Scholarship aimed at helping small Pacific island nations with capacity building in economic development areas.
“This research will help energy providers and the government. It assists in identifying potential wind source sites and their wind capacity. We have to site wind farms in the areas with highest capacity, to produce the most electricity,” says Kunal.
Fijian weather varies between years, where atmospheric and synoptic systems such as tropical storms and the Southeast trade winds affect wind patterns among others. Because of this, Kunal needed to model decade long wind resource maps and create long-term wind data to understand wind variability and model wind farms for utility-scale wind power applications in Fiji. These didn't exist in Fiji, so he combined analysis data from General Circulation Models with the Weather Research and Forecasting (WRF) model to fill the gaps.
“Fiji weather station data is available, but only between three-to-eight years, depending on location. And not all these locations are located in high wind resource areas. If you want to build large-scale wind farms to support utility-scale wind power generation, you need high-resolution wind-resource maps and a minimum of 10 years uninterrupted wind data to inform your location scouting and wind farm modelling,” says Kunal. “We used a methodology called dynamical downscaling, whereby coarse resolution output from an analysis using a General Circulation Model (GCM) are used as initial and boundary conditions to drive a regional numerical model (WRF) to simulate atmospheric parameters via nesting, considering the local conditions to simulate the wind resource at a high-resolution of 1 km × 1 km.”
Kunal’s research needed the Weather Research and Forecasting (WRF) model to execute the high-resolution mesoscale wind-resource modelling. The WRF model solves the non-linear partial differential equations for the conservation of mass, momentum, energy and moisture phases to simulate the atmospheric parameters including wind resource parameters at heights of interest. To use the state-of-the-art WRF model, installation of the model and its support data libraries to NeSI’s Mahuika supercomputer was required to successfully run the WRF model. NeSI's support team helped Kunal bring these programs together to run the full model.
“I met with NeSI staff because I had problems installing my model on Mahuika. My model had specific versions of support programs it needed to run. I was having problems linking my model directory to the specified required supporting software programs. NeSI support helped me line everything up so the model could run efficiently on Mahuika.”
Kunal’s mesoscale modelling research study using the WRF model found 40 new wind farms sites in Fiji [1], which includes Rakiraki, Nabouwalu and Udu that were previous found by Kunal via using ground-based measured wind data from automatic weather stations via microscale modelling using the industry standard WAsP model [2]. These 40 sites are well spread, making them ideal for covering the power demand on Fiji’s two largest islands.
“The numerical weather prediction model would be impossible to run on a standard desktop computer for a decade-long wind-resource assessment study. It’s covering the mesoscale, between a few kilometres to hundreds of kilometres across all of Fiji at 1 km × 1 km grid resolution. We needed high-performance computing to run model simulations for a decade-long at this resolution, so NeSI has been vital in that respect,” says Kunal.
Fiji's government, private sector, industry and international investors can use Kunal's research output to build wind farms to power the country. While Kunal identified the 40 most valuable sites, his work with NeSI created a high-resolution wind resource map and long-term time series wind data covering all of Fiji. It will help the islands move away from their reliance on petroleum exports and into a greener future.
Figure 1 presents the 55-metre elevation mean wind speed map of Fiji at 1 km × 1 km grid resolution. Wind speed greater than 6.4 m/s (NREL, Wind Power Class 3) are suitable for utility-scale wind power applications.
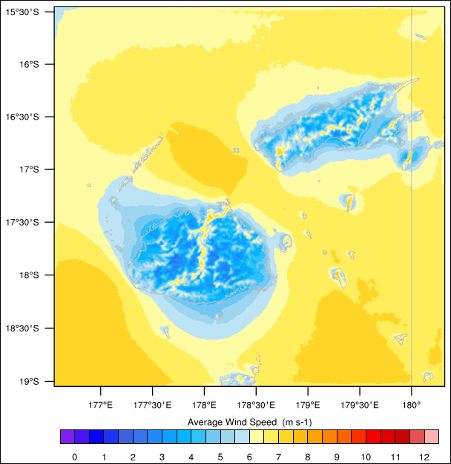
References:
[1] Dayal, K.K., Bellon, G., Cater, J.E., Kingan, M.J. and Sharma, R.N. (2021). High-resolution mesoscale wind-resource assessment of Fiji using the Weather Research and Forecasting (WRF) model. Energy, 232, 2021.
[2] Dayal, K.K., Cater, J.E., Kingan, M.J., Bellon, G.D. and Sharma, R.N. (2021). Wind resource assessment and energy potential of selected locations in Fiji. Renewable Energy, 172, 219-237.
Do you have an example of how NeSI support or platforms have supported your work? We’re always looking for projects to feature as a case study. Get in touch by emailing support@nesi.org.nz.