Helping refine Parkinson’s disease analysis
Around 10,000 New Zealanders suffer from Parkinson’s disease. This degenerative disorder of the central nervous system does not only limit a patient’s movement, says Dr Daniel Myall, a computational neuroscientist at the New Zealand Brain Research Institute in Christchurch. “Many, though not all, Parkinson’s patients eventually also develop cognitive decline, which then becomes the most burdensome aspect of this progressive condition.”
There is currently no treatment for cognitive decline in Parkinson’s disease, so there’s a need to detect those who are at high risk of cognitive decline so they can be targeted in forthcoming therapeutic trials.
Myall is part of a team that has a database of brain scans and longitudinal cognitive measures of people with Parkinson’s disease at its disposal, but analysing this data in order to extract useful measures of brain structure and function – and then combining these into a model of the probabilistic risk of cognitive decline – is computationally demanding.
Access to the New Zealand eScience infrastructure (NeSI) presents Myall and his team with an opportunity to conduct its research faster. “NeSI provides resources with enough processing power and memory to enable pre-processing and model formation to be performed in a reasonable time – days as opposed to months,” says Dr Myall.
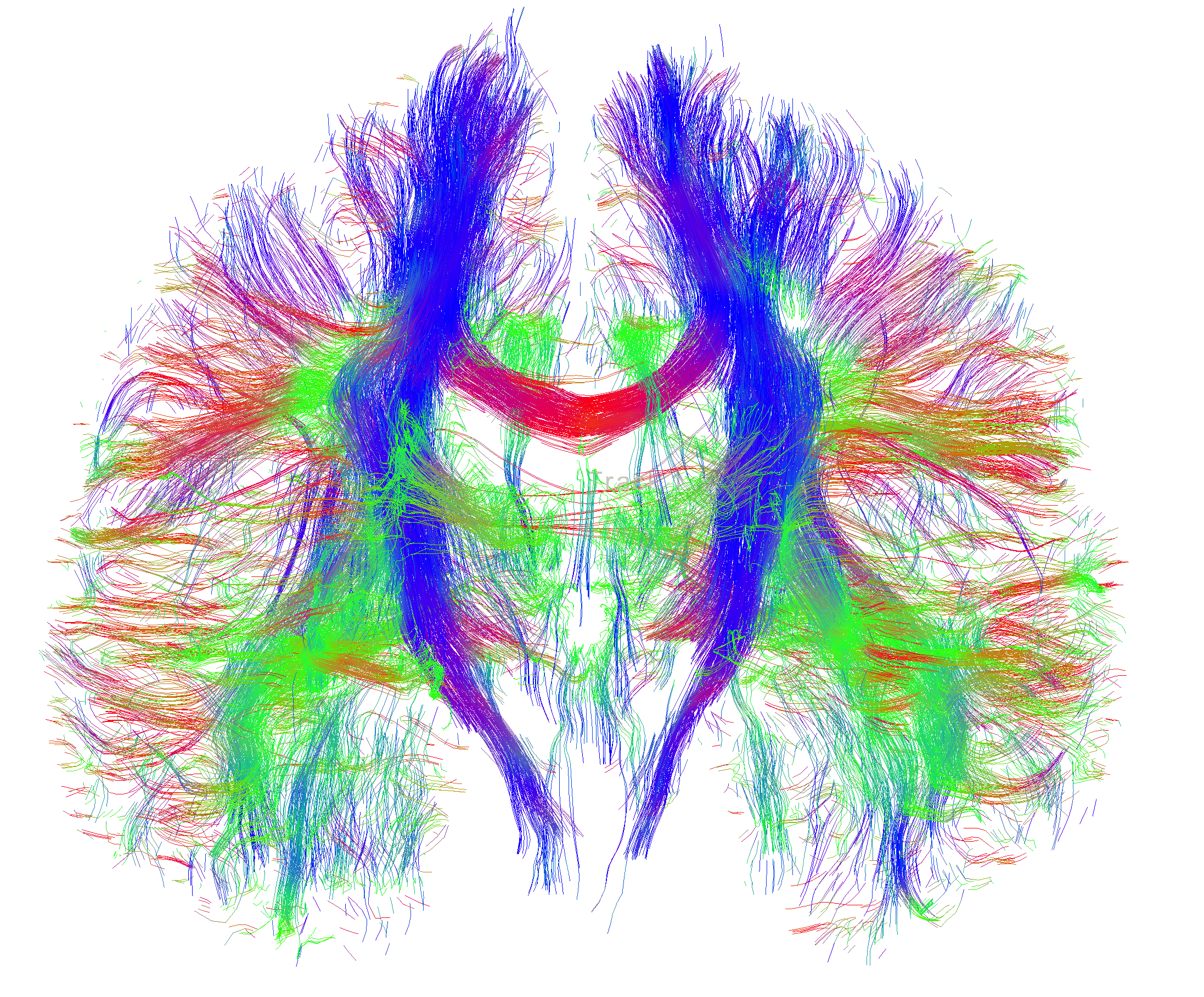
Calibrating prediction models
Myall’s model of cognitive decline is trained on data from 170 individuals with multiple measurements over time. Those predictions will then be validated. “Over the next two years many of these individuals will be followed up. Success is determined by how accurate the predictions are compared to the actual change in cognition.”
The study should provide a clear indication of the ability to predict cognitive change based upon modern brain imaging techniques, says Myall. “The primary methodology is to use existing magnetic resonance imaging (MRI) analysis packages to extract measures of brain structure and function and then use Bayesian techniques to optimally learn from this what measures are most useful for predicting future cognition.”
Myall trained in mathematics before he moved into medicine, and he also has extensive experience in computing and programming. For his PhD Myall developed a virtual environment platform and used it to examine motor adaptation in Parkinson’s disease.
Myall says his main computational challenges now relate to adapting the MRI analysis software to work well on NeSI, and implementing the Bayesian learning algorithms to work efficiently in a high performance computing environment.
Members of NeSI’s computational science team have provided Myall with technical assistance. This has included integrating his software with IBM Tivoli Workload Scheduler LoadLeveler. LoadLeveler is a parallel job-scheduling system that allows users to run more jobs in less time by matching the processing needs and priority of each with available computational resources to maximise resource use.
“A probabilistic analysis of the major axonal pathways in the brain takes around 18 hours on a single core,” says Myall. “This can be efficiently parallelised, and on a 32-core node runs in 35 minutes. This makes it much easier to iteratively refine and improve the analysis, as we don’t have to wait days for a single analysis or months to analyse the entire collection of data.”
Once Myall’s software is fully integrated with LoadLeveler, Myall hopes to be able to determine changes in the pathways of over 300 brains in various states of cognitive impairment. He and his team are continuously learning from the difficulties associated with having many more predictors than individuals, as well as compensating for missing data and making the algorithms run more efficiently.
“This makes it much easier to iteratively refine and improve the analysis, as we don’t have to wait days for a single analysis or months to analyse the entire collection of data.”
— Dr Daniel Myall, computational neuroscientist, New Zealand Brain Research Institute“
If we were to start the entire project again there are several things that would be improved on the data collection side,” says Myall, “as many of the computational difficulties arise due to complexities with the data.”
Myall would recommend NeSI to his science research peers because access to the HPC network means they don’t have to buy and maintain their own workstations and it allows large computational tasks to be completed quickly when required. “I’ve always found the NeSI support staff helpful when I’ve required assistance,” he says.
While it’s currently still in its implementation phase, Myall’s research project may in the future be commercialised. “It’s possible that our predictive models of cognitive decline risk may be of commercial interest to pharmaceutical companies who wish to trial potential treatments,” says Myall. “This will allow sample enrichment with individuals who are at greatest risk of cognitive decline and will make clinical trials more effective.”
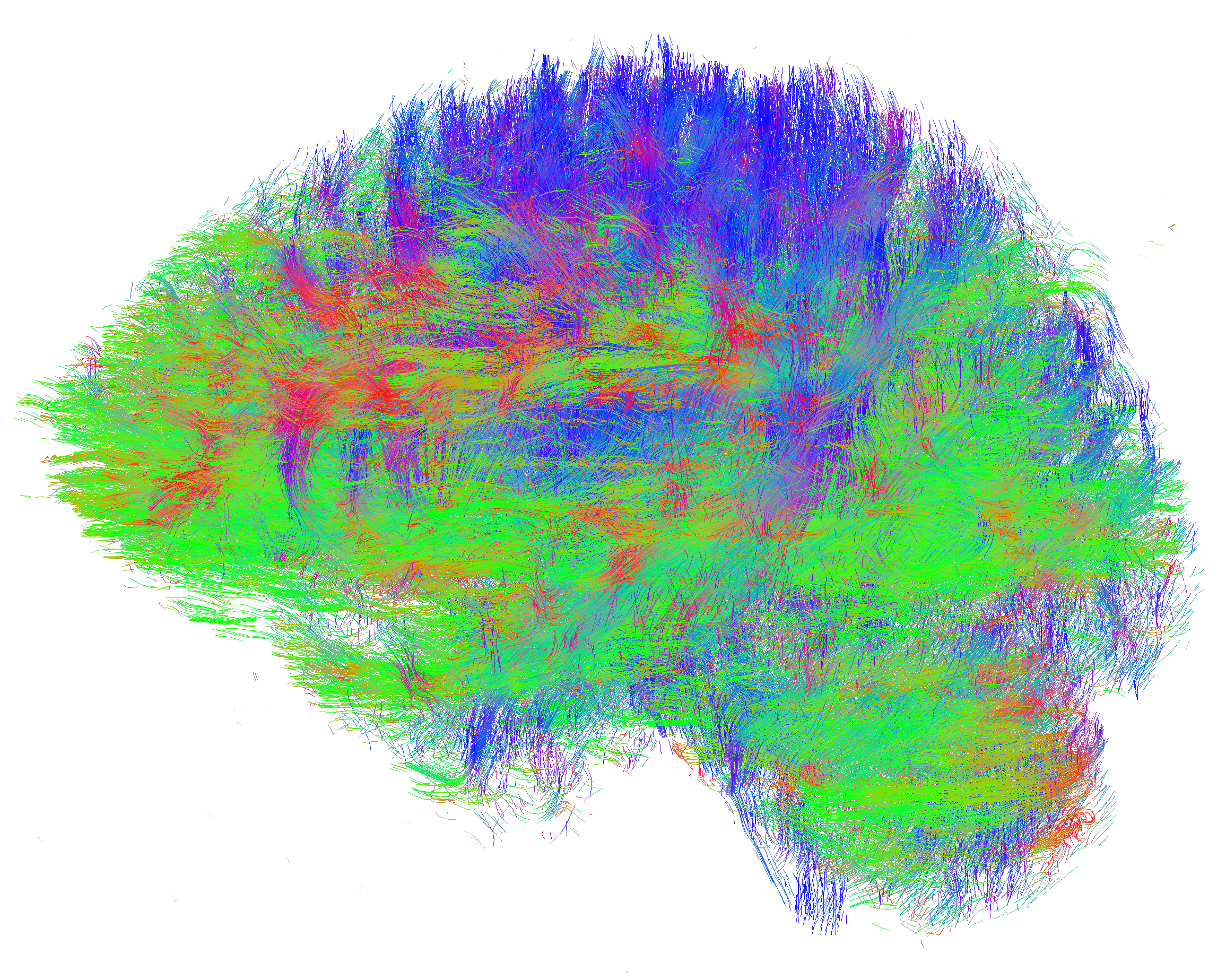